Machine Learning
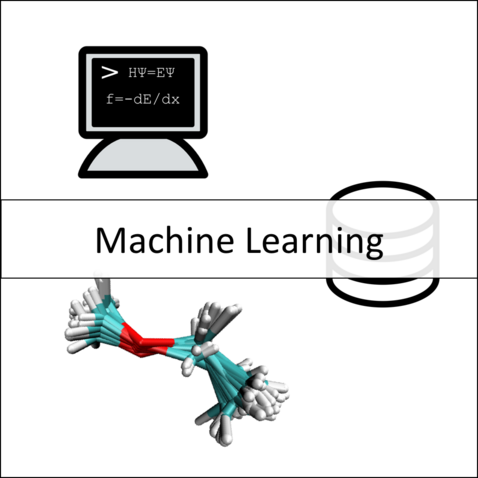
Electronic structure theory is in principle the most attractive framework for making predictions in chemistry. Unfortunately, the complexity of the underlying equations of quantum mechanics limits the applicability of this rigorous approach, both with respect to the size of the systems that can be considered and in the number of calculations that can be performed, e.g. in a screening study. Our interest in complex reaction networks with millions of potentially important elementary steps has lead us to consider alternative approaches, which can be applied on such a large scale.[1]
Here, machine learning (ML) has emerged as a promising new tool in recent years. Essentially, ML takes advantage of the fact that, although the equations of quantum mechanics are highly nonlinear and complex, the connection between the total energy of a molecule and its geometry and composition (i.e. the potential energy surface) tends to be quite smooth and systematic. If this connection can be learned, complex quantum simulations can be circumvented entirely. In this context, we have also explored the importance of ensuring exact physical constraints like size-extensivity in ML models.[2]
In a second project, we are exploring the use of ML as an integrated part of electronic structure theory, rather than its replacement. In this context, we have developed a ML model that infers the coupled cluster correlation energy based on wavefunctions from second-order perturbation theory, drastically reducing the computational cost of high quality calculations.[3]