Concept and objectives
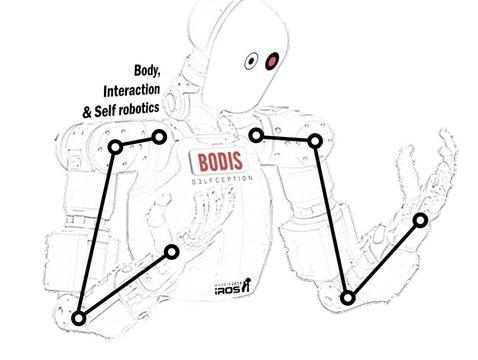
Humans learn and later continuously update models of their bodies and the world by means of interaction during their whole life and are able to discern between their own body and self-produced actions and other entities in their surroundings. Thereby, humans can seemingly effortlessly cope with complex unstructured environments using predictors and interact with other entities and humans inferring their physical (location and dynamics), emotional, and mental state. Although we are still far from this scenario in robotics, promising models and methods appear every year with great potential for real world applications. However, there are still barriers that should be overcome, such as the lack of proper experimental evaluation, oversimplification of the models, scalability or generalization. Usually, the complexity of the learning and modelling schemes makes difficult to envisage its utility for end-user areas like robot companions, human-robot interaction or industrial applications. Hence, this workshop discusses the utility and applicability of current computational models that allow robots to learn their body and interaction models to deploy robust adaptive physical interaction by exploiting the learnt prediction capabilities. Drawing on recent developments in embodied artificial intelligence and robotic sensing technology, engineering and bio-inspired solutions will be presented to solve challenging problems, such as automatic multimodal self-calibration (e.g. soft robots), interactive perception and learning (e.g. causal inference), safe human-robot interaction (e.g. peripersonal space learning) and safe interaction in complex human environments (e.g. self/other distinction).
The main objectives of the workshop are:
- To present the utility and advantages of autonomous learning of body, interaction, and self models in robotics, and their underlying mechanisms as critical processes for human-robot interplay, interaction in unstructured environments and tool use.
- To bring together roboticists that started theorizing about this approach with new researchers from the field of embodied AI and robotics, in order to present and discuss the main developments, benefits and current challenges for deploying a new generation of multisensory robots that learn from interaction.
Particularly, four important pillars will be addressed in detail. (I) Body: sensors and actuators to provide successful body learning capabilities. (II) Exploration: methods to efficiently sample information from the body and the environment. Different complementary methods have been proposed, such as neural oscillators, intrinsic motivation, goal directed movements, social and imitation driven exploration. (III) Learning: sensorimotor integration algorithms to encode body patterns and enable prediction. Promising connectionist approaches are multiple timescales recurrent neural networks, Hebbian-based and deep learning like restricted Boltzmann machines; Bayesian inference solutions, such as variational Bayes and predictive processing; and high-dimensionality regressors like Gaussian process, locally weighted projection regression or infinite experts. (IV) Memory: the model, the parameters or the priors are stored during interaction for future predictions. The memory highly depends on the learning algorithm. Some potential approaches are parameterization, Gaussian factorizations, RNN layers with different temporal dynamics, episodic memories and associative networks.